Guide to
RWD and RWE
Real-World Data (RWD) and Real-World Evidence (RWE) are terms commonly used in the pharmaceutical space. In general, pharmaceutical experts have a pretty good understanding of where the data comes from: anywhere but clinical trials.
Now, that doesn’t seem very specific, does it?
The goal of this guide is to shed a bit more light into it and explain the basic definitions and terminology related to RWD and RWE, see what the difference is, and look at some examples where the data comes from, if not clinical trials.
Definition of Real-World Data (RWD)
Real-World Data (RWD) is data that is relevant to a patient’s health status or data related to the delivery of healthcare.
RWD can come from different sources such as electronic health records (EHR), medical claims and billing data, patient-generated data such as wearables and health apps, and data from product registries and disease registries.
Definition of Real-World Evidence (RWE)
Real-World Evidence (RWE) is information derived from analysis of RWD that can be used in the regulatory processes and private or public decision making related to a medicinal product, medical device or any other kind of treatment.
RWE is clinical evidence obtained from the analysis of RWD that provides information about usage, risks, and benefits of the treatment method derived from sources other than traditional randomized clinical trials (RCT).
Difference between RWD and RWE
For example, RWE is proof that an orphan drug is effective and safe even though the patient population in the country is very small.
RWE is data from the field that can be used to decide that a drug’s indication can be expanded based on different complex scenarios from real-life treatments of people outside of the controlled environment of clinical trials.
RWE is a justification to cover the costs of a new treatment when the endpoints of the clinical trial show only minor differences to established products.
RWD is the data that is used to build this evidence, justification, or other arguments.
Features of RWE
RWE differs from clinical evidence because RWD is not collected for the purpose of generating evidence.
- RWE is prospective or retrospective
- RWE is not randomized
- RWE is heterogeneous
- RWE may or may not have a control arm
This means that you cannot design plans for the collection of RWD because if you do that, you are already conducting a clinical trial. When the RWD is retrieved from registries and databases, you need to ensure the information is randomized, anonymized and cleaned. Because you cannot plan the design for the collection of RWD.
By default, there is no control arm and if some type of control is needed, it needs to be a randomized clinical trial, or it should be created from another dataset. That said, RWD can be used as an external control arm for a clinical study.
1) Pre-clinical research
2) Clinical trials & studies
3) Pharmacovigilance
4) Market access, launch, and post-launch activities
Use of RWE
There is no definitive answer to this and it is hard to pinpoint a specific stage of a product life-cycle when RWE is or can be used. RWE is information and the pharmaceutical industry aims to make informed decisions at all times during the life-cycle of the product.
If we wanted to be very simplistic, we could just say RWE has no categories as it is used throughout a product’s lifecycle from the very beginning and as long as it’s available to patients (or even after).
But we can make an effort and break down the use of RWE to clarify to readers where it is typical to see RWE in use. Acknowledging the bias of RemedyBytes’s current activities, we have divided the key types of use-cases for RWE into 4 categories.
1) Pre-clinical research
2) Clinical trials & studies
3) Pharmacovigilance
4) Market access, launch, and post-launch activities
Pre-clinical Research
RWE can be used to guide the product pipeline and portfolio strategy and to inform the product development and clinical development. In these cases, the use of RWE is intimately tied to the product development strategy.
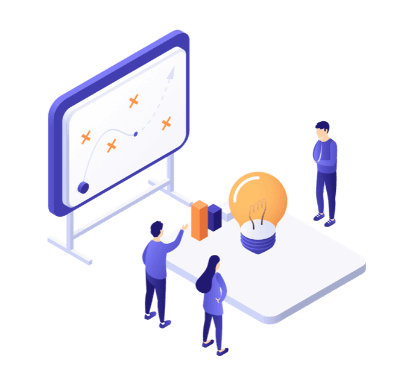
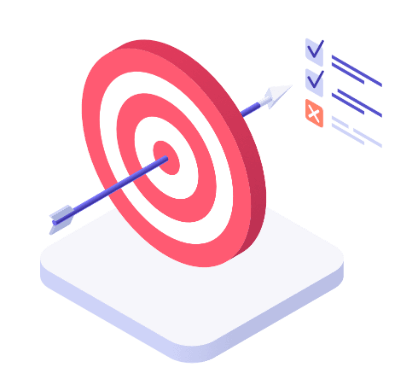
RWD can be one of the data sources when developing Target Product Profiles (TPPs), which help guide internal decisions throughout the product development process.
As a side note, the term real-world insight (RWI) is sometimes used to describe the analysis of RWD for the purpose of internal decision making at a pharmaceutical company or medical device manufacturer.
RWD can be analyzed to provide insights into the targeted indication by refining available estimates of disease prevalence and incidence, including temporal trends, to help predict how the total patient population may change over time.
Clinical Trials & Studies
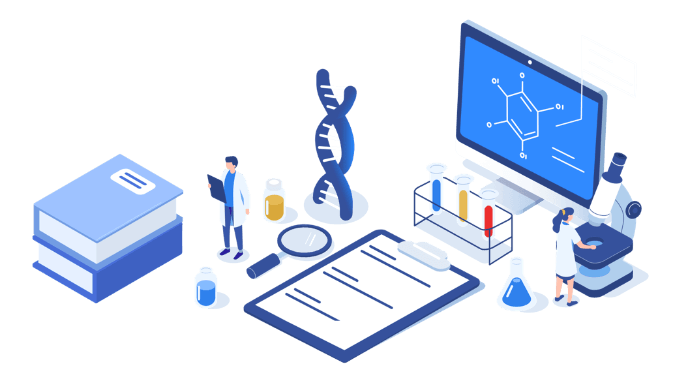
Medicinal product developers use RWD and RWE to support clinical trial designs (e.g., large simple trials, pragmatic clinical trials) and observational studies to generate innovative, new treatment approaches.
The controlled nature of a randomized controlled trial offers advantages in evidence generation thanks to the standard methods to reduce bias (like randomization and blinding) and comprehensive measurement of outcomes to demonstrate efficacy against both active and placebo controls but RCTs do not accurately reflect real-world circumstances under which patients are treated. That is why there is often a need for observational studies to support additional evidence generation.
One area of use for RWE is clinical practice, where it can support regulatory decisions in situations where “traditional” clinical data is difficult and/or too expensive to collect. This “may be the case” with rare diseases or highly personalized treatments such as oncological products, as an example.
Pharmacovigilance
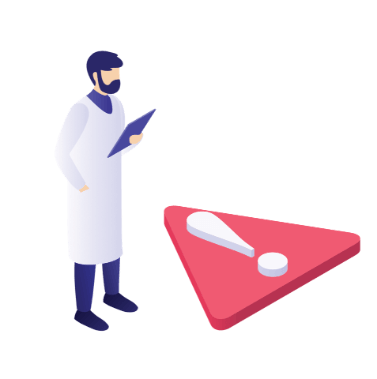
RWE is also used in medical post-authorization and rare diseases where it is used especially in post-authorization safety studies (PASS) and post-authorization efficacy studies (PAES).
RWE can be used in other pharmacovigilance activities such as safety signal detection, extending and restricting indications, monitoring, and reporting of medicinal product risk-benefit ratio (PSUR / PBRER), and withdrawal of marketing authorization.
Market Access, Launch and Post-launch Activities
The healthcare community and life science industry is using Real-world evidence to support coverage decisions, market authorizations and pricing decisions especially for new and innovative treatments. These activities can be packaged under the term “Market Access”.
RWE can be also used to expand the indication profile of a product. RWE is also used to develop guidelines and decision support tools for use in clinical practice. Key factor is using RWE in demonstrating the efficacy of a treatment compared to other treatment alternatives.
In the post-marketing phase, the pharmaceutical companies and medical device manufacturers can use RWD and RWE to understand their product positioning, cost-efficiency, dynamic market changes, comparisons to alternative products and to comply with regulatory demands.
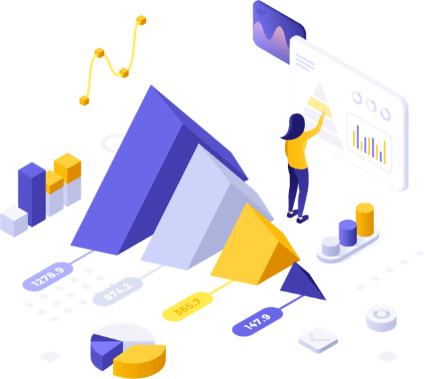
Sources of RWD
Now that we know the definition and uses of RWD and RWE, let’s dig deeper into where RWD comes from and how to generate RWE.
Below, we list some of the most commonly used sources of RWD and the ways it has been used to produce RWE.
There are several different data sources to go through to identify the correct right one(s) for your specific situation. The data does not have to come from one place only, like a hospital’s electronic medical record (EMR) system, and it can be a combination of patient data and for example the national registry for cause of death.
The examples here are probably the most common data sources for RWD but we want to highlight that these are not the only sources of RWD which is the great thing about RWD: new data collection methods and data sources are popping up all the time and the quality and amount of data keeps increasing. Newer data sources not described here in detail are for example genomic data from biobanks, biopsies and other pathology tests, diagnostic imaging, social determinants of health (SDoH), cancer organoids, and patient-derived xenografts (PDXs).
Hospital Databases
Depending on the country, retrieving data which originates from hospital databases is relatively “cheap and fast”.
For example, in the UK it can take about 4 – 6 months to fetch data from a database for a single hospital or group of connected hospitals – also known as a Hospital Information System, e.g. UK Hospital Episode Statistics (HES) database. The cost of data retrieval depends on the administrative costs of the registry or database holder.
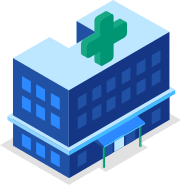
The “cheap and fast” here is meant to be sarcastic.
In the current world where data is being collected about everything into electronic databases and APIs are a standard (albeit relatively unknown one), it should not take months of waiting and days of administrative work to retrieve data.
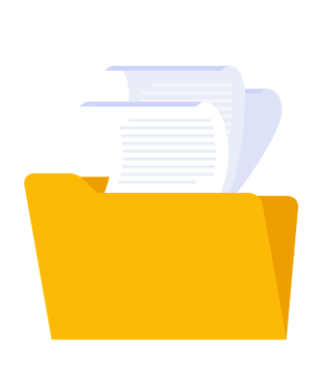
The hospital databases can include inpatient diagnoses, procedures performed, medical devices and drugs prescribed and dispensed, equipment and supply fees, imaging, inpatient laboratory results, discharge location, etc. Additional information can include for example the costs of the treatment, medication or supplies used.
The obvious limitation of data collected in a hospital environment is that the full details are available on inpatient stay only. If the records are from an outpatient facility, there might be only partial data available.
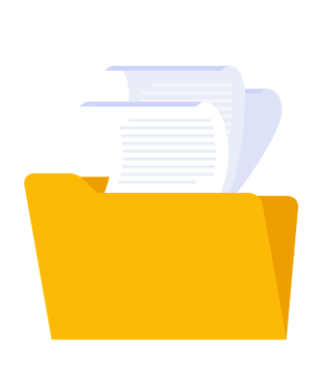
Administrative and Reimbursement Claims Databases
The Nordics are in many ways the frontrunners in the quality and availability of reimbursement and other healthcare data for secondary and statistical use.
Local authorities are constantly developing their services and in some cases good quality real-world data can be accessed in a matter of weeks, or even through a direct API. And the price of retrieving the data would usually be a fraction of the costs described above for the US.
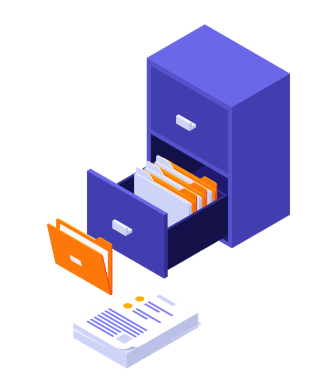
Mobile Health Data – Wearables and Apps
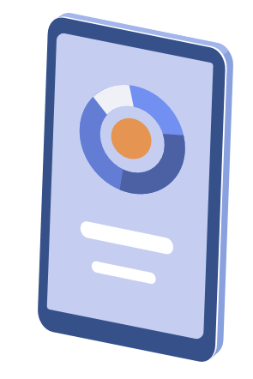
Data collected with mobile devices can be useful to understand functional status, quality of care, adherence, and treatment preference of the patient. However, some of the data reliability can be limited because the reliability is dependent on patient understanding and recall.
The amount of data from mobile is limited by the participation rates. But, if you look at how easily most of us opt in to share our information collected by apps, phones and watches, and how the big tech companies are rushing to the health industry and life sciences, mobile health data will be or most likely already is the largest dataset of the data sources described here. The big question is the retrieval of that data due to the private actors and data privacy issues.
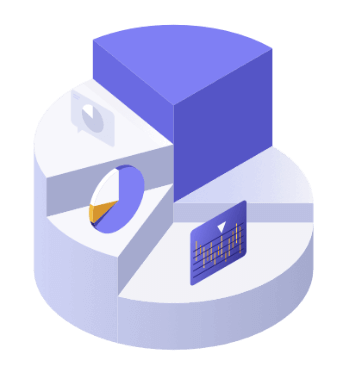
Electronic Medical Records
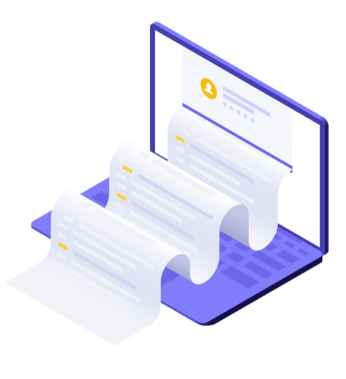
Depending on the country or region and the local healthcare system, electronic medical records can be more expensive to acquire, and the process might take longer, for example 6 – 9 months.
Electronic medical records contain data used to capture and record an accurate and complete patient health record. An electronic medical record is the digital version of a paper chart.
The strength of EMRs is that they include full details of patient-healthcare professional interaction, including laboratory results, pathology, disease staging, disease severity,etc. EMRs usually contain both structured data (age, gender, location, dates, etc.) and unstructured data (treatment notes, patient-reported outcomes, lifestyle, behavior, risk factors, etc.) In some situations, the EMRs also include administrative and billing data.
If the electronic medical records contain structured data and data management practices at the source organization are good, the extraction of desired information can be relatively quick.
Unstructured data requires natural language processing (NLP) or some other advanced extraction method to put it into usable form. This area of linguistics and artificial intelligence is advancing quickly and one of the key factors for the growth of business related to NLP is the potential for structuring health data. Based on the recent developments with OpenAI’s tools, one can be hopeful that there is soon a large new dataset available from old unstructured EMRs.
Electronic medical records are often limited to the inpatient and outpatient setting. This means that EMRs may miss encounters outside the electronic medical records network. In regards to the use of medicinal products, EMRs reflect intent to prescribe rather than patients going to the pharmacy to get the prescribed medication or actually using the product.
Another downside of electronic medical records is that, unfortunately, there is little to none harmonization between the systems used to create and manage EMRs among hospitals, hospital regions, healthcare regions, cities or countries. When combining data from different areas, data cleaning and manipulation is required.
EMRs can be used to create RWE related to physician prescribing patterns, treatment effectiveness, natural history of disease and course of treatment, risk factors/predictors of disease outcomes, development of standard-of-care cohort for comparison with single-arm clinical trial, etc.
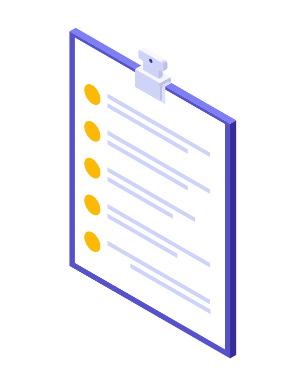
Surveys
Surveys, focus groups, and interviews with patients, caregivers, and healthcare providers (HCPs) are a relatively cheap data source. Planning, organizing and compiling data from a survey takes about 2 – 3 months with an established panel.
Patient surveys can offer a valid and reliable method for collecting patient-relevant data that supplement data from other sources and for gathering new information that is not available from other data sources. Surveys can be useful to understand functional status, quality of care, adherence, treatment preference etc.
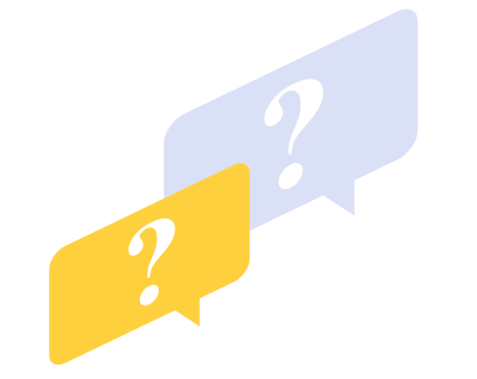
The data reliability of surveys is dependent on patient understanding and recall. Based on the surveying method, the participation rates can be low and this can skew the reliability of the results. The survey wording can be a source of bias.
Like mobile e-health data, surveys can be used to calculate health-related quality of life values for patients and caregivers and to capture patient-reported outcome measures.
Systematic Literature Review
Literature review is probably the cheapest data source for RWE. It can also be the fastest to acquire. Depending on the sources, tools and staff, the process takes 1 – 6 months. Literature review would include the review of published secondary data using various databases.
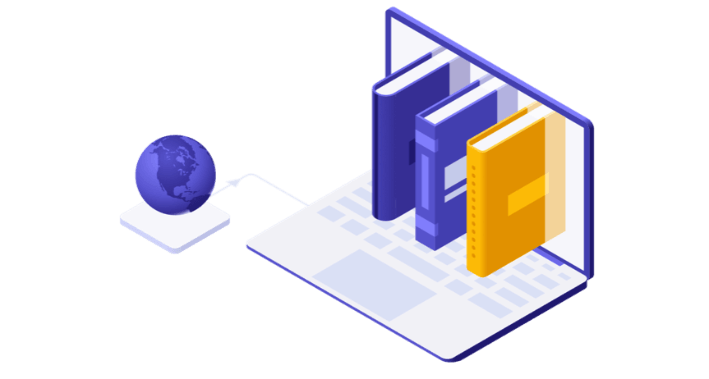
Literature review can be used to study different questions. Literature review can be a source of data for economic models and useful for burden-of-illness studies. An obvious limitation of systematic literature review is that the ability to answer questions is based on availability of published data and the variability in study design and data recording can create challenges when trying to combine information from different articles.
Setting up a new Registry
If there are now usable databases or registries available and clinical trials are difficult or too expensive to perform, creating a new registry is an option. However, setting up a registry is also expensive and it can take about 12–18 months to do.
Registry is an organized system that uses observational study methods to collect uniform data (clinical and other) to evaluate specified outcomes for a population defined by a particular disease, condition, or exposure, and that serves a predetermined scientific, clinical, or policy purpose.
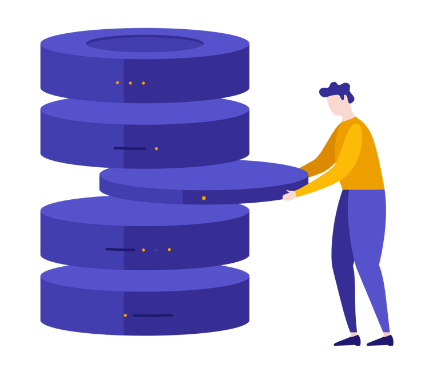
Most important part about registry studies is that they provide more information on patient subpopulations that are not necessarily included in clinical trials and they can provide a possibility for long-term follow-up. Registry design can be customized to suit one’s needs.
This is also the main challenge: in traditional settings, the usefulness of collected data for research purposes depends on advanced planning and strong vision for the registry. Patient enrollment for the registry can be challenging depending on the disease area. The study sites may not collect all the requested data or the data quality can be low. In short, building a new registry is a lot of work, but sometimes it is worth it.
Summary
RWD is data that is relevant to a patient’s health status or it can be data related to the delivery of healthcare. RWE is information derived from analysis of RWD that can be used in the regulatory processes and private or public decision making related to a medicinal product, medical device or any other kind of treatment.
It should be noted that there are several different definitions for both and they vary between regions and countries. Also the use of RWE varies a bit based on the authority and as this is a relatively new area in the regulatory space, even the definitions can be updated.
RWE is prospective or retrospective, heterogeneous, not randomized and usually does not have a control arm but it can be used as a control arm for clinical trials. There are several different data sources, and the quality and usability of the data depends heavily on the data source. This means that it is important to know what kind of data is available and what can be done with it.
While this page is called the “The Guide to RWD and RWE” we have to admit it does not contain all the information available. We’ll publish additional guides for different aspects including regional and regulatory analysis of RWE and go back to the basic definitions regularly to see what has changed.
Remedybytes 2024 | Privacy policy